OpenAI brings GPT-4.1 and 4.1 mini to ChatGPT — what enterprises should know
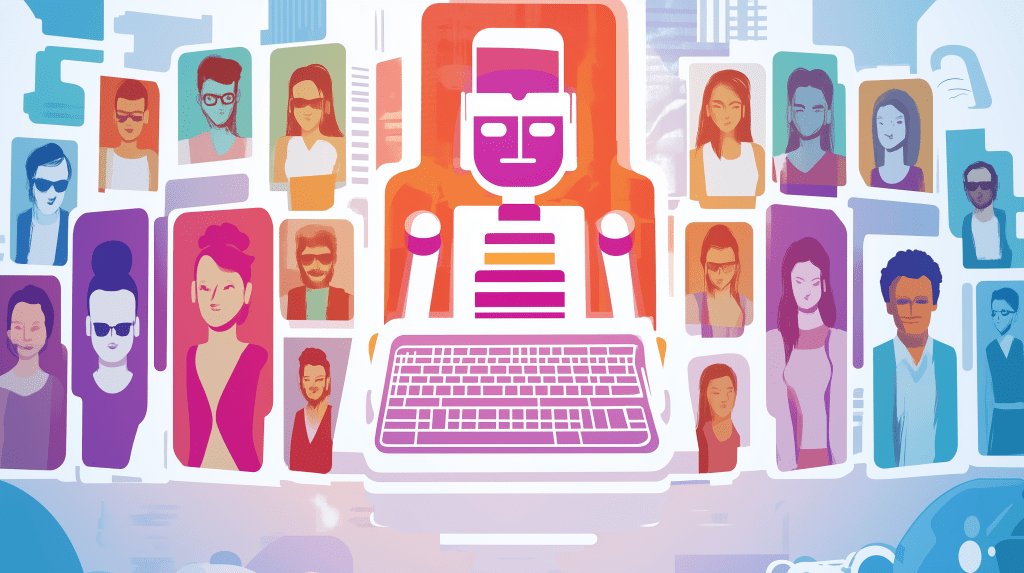
Join our daily and weekly newsletters for the latest updates and exclusive content on industry-leading AI coverage. Learn More
OpenAI is rolling out GPT-4.1, its new non-reasoning large language model (LLM) that balances high performance with lower cost, to users of ChatGPT. The company is beginning with its paying subscribers on ChatGPT Plus, Pro, and Team, with Enterprise and Education user access expected in the coming weeks.
It’s also adding GPT-4.1 mini, which replaces GPT-4o mini as the default for all ChatGPT users, including those on the free tier. The “mini” version provides a smaller-scale parameter and thus, less powerful version with similar safety standards.
The models are both available via the “more models” dropdown selection in the top corner of the chat window within ChatGPT, giving users flexibility to choose between GPT-4.1, GPT-4.1 mini, and reasoning models such as o3, o4-mini, and o4-mini-high.
Initially intended for use only by third-party software and AI developers through OpenAI’s application programming interface (API), GPT-4.1 was added to ChatGPT following strong user feedback.
OpenAI post training research lead Michelle Pokrass confirmed on X the shift was driven by demand, writing: “we were initially planning on keeping this model api only but you all wanted it in chatgpt 🙂 happy coding!”
OpenAI Chief Product Officer Kevin Weil posted on X saying: “We built it for developers, so it’s very good at coding and instruction following—give it a try!”
An enterprise-focused model
GPT-4.1 was designed from the ground up for enterprise-grade practicality.
Launched in April 2025 alongside GPT-4.1 mini and nano, this model family prioritized developer needs and production use cases.
GPT-4.1 delivers a 21.4-point improvement over GPT-4o on the SWE-bench Verified software engineering benchmark, and a 10.5-point gain on instruction-following tasks in Scale’s MultiChallenge benchmark. It also reduces verbosity by 50% compared to other models, a trait enterprise users praised during early testing.
Context, speed, and model access
GPT-4.1 supports the standard context windows for ChatGPT: 8,000 tokens for free users, 32,000 tokens for Plus users, and 128,000 tokens for Pro users.
According to developer Angel Bogado posting on X, these limits match those used by earlier ChatGPT models, though plans are underway to increase context size further.
While the API versions of GPT-4.1 can process up to one million tokens, this expanded capacity is not yet available in ChatGPT, though future support has been hinted at.
This extended context capability allows API users to feed entire codebases or large legal and financial documents into the model—useful for reviewing multi-document contracts or analyzing large log files.
OpenAI has acknowledged some performance degradation with extremely large inputs, but enterprise test cases suggest solid performance up to several hundred thousand tokens.
Evaluations and safety
OpenAI has also launched a Safety Evaluations Hub website to give users access to key performance metrics across models.
GPT-4.1 shows solid results across these evaluations. In factual accuracy tests, it scored 0.40 on the SimpleQA benchmark and 0.63 on PersonQA, outperforming several predecessors.
It also scored 0.99 on OpenAI’s “not unsafe” measure in standard refusal tests, and 0.86 on more challenging prompts.
However, in the StrongReject jailbreak test—an academic benchmark for safety under adversarial conditions—GPT-4.1 scored 0.23, behind models like GPT-4o-mini and o3.
That said, it scored a strong 0.96 on human-sourced jailbreak prompts, indicating more robust real-world safety under typical use.
In instruction adherence, GPT-4.1 follows OpenAI’s defined hierarchy (system over developer, developer over user messages) with a score of 0.71 for resolving system vs. user message conflicts. It also performs well in safeguarding protected phrases and avoiding solution giveaways in tutoring scenarios.
Contextualizing GPT-4.1 against predecessors
The release of GPT-4.1 comes after scrutiny around GPT-4.5, which debuted in February 2025 as a research preview. That model emphasized better unsupervised learning, a richer knowledge base, and reduced hallucinations—falling from 61.8% in GPT-4o to 37.1%. It also showcased improvements in emotional nuance and long-form writing, but many users found the enhancements subtle.
Despite these gains, GPT-4.5 drew criticism for its high price — up to $180 per million output tokens via API —and for underwhelming performance in math and coding benchmarks relative to OpenAI’s o-series models. Industry figures noted that while GPT-4.5 was stronger in general conversation and content generation, it underperformed in developer-specific applications.
By contrast, GPT-4.1 is intended as a faster, more focused alternative. While it lacks GPT-4.5’s breadth of knowledge and extensive emotional modeling, it is better tuned for practical coding assistance and adheres more reliably to user instructions.
On OpenAI’s API, GPT-4.1 is currently priced at $2.00 per million input tokens, $0.50 per million cached input tokens, and $8.00 per million output tokens.
For those seeking a balance between speed and intelligence at a lower cost, GPT-4.1 mini is available at $0.40 per million input tokens, $0.10 per million cached input tokens, and $1.60 per million output tokens.
Google’s Flash-Lite and Flash models are available starting at $0.075–$0.10 per million input tokens and $0.30–$0.40 per million output tokens, less than a tenth the cost of GPT-4.1’s base rates.
But while GPT-4.1 is priced higher, it offers stronger software engineering benchmarks and more precise instruction following, which may be critical for enterprise deployment scenarios requiring reliability over cost. Ultimately, OpenAI’s GPT-4.1 delivers a premium experience for precision and development performance, while Google’s Gemini models appeal to cost-conscious enterprises needing flexible model tiers and multimodal capabilities.
What It means for enterprise decision makers
The introduction of GPT-4.1 brings specific benefits to enterprise teams managing LLM deployment, orchestration, and data operations:
- AI Engineers overseeing LLM deployment can expect improved speed and instruction adherence. For teams managing the full LLM lifecycle—from model fine-tuning to troubleshooting—GPT-4.1 offers a more responsive and efficient toolset. It’s particularly suitable for lean teams under pressure to ship high-performing models quickly without compromising safety or compliance.
- AI orchestration leads focused on scalable pipeline design will appreciate GPT-4.1’s robustness against most user-induced failures and its strong performance in message hierarchy tests. This makes it easier to integrate into orchestration systems that prioritize consistency, model validation, and operational reliability.
- Data engineers responsible for maintaining high data quality and integrating new tools will benefit from GPT-4.1’s lower hallucination rate and higher factual accuracy. Its more predictable output behavior aids in building dependable data workflows, even when team resources are constrained.
- IT security professionals tasked with embedding security across DevOps pipelines may find value in GPT-4.1’s resistance to common jailbreaks and its controlled output behavior. While its academic jailbreak resistance score leaves room for improvement, the model’s high performance against human-sourced exploits helps support safe integration into internal tools.
Across these roles, GPT-4.1’s positioning as a model optimized for clarity, compliance, and deployment efficiency makes it a compelling option for mid-sized enterprises looking to balance performance with operational demands.
A new step forward
While GPT-4.5 represented a scaling milestone in model development, GPT-4.1 centers on utility. It is not the most expensive or the most multimodal, but it delivers meaningful gains in areas that matter to enterprises: accuracy, deployment efficiency, and cost.
This repositioning reflects a broader industry trend—away from building the biggest models at any cost, and toward making capable models more accessible and adaptable. GPT-4.1 meets that need, offering a flexible, production-ready tool for teams trying to embed AI deeper into their business operations.
As OpenAI continues to evolve its model offerings, GPT-4.1 represents a step forward in democratizing advanced AI for enterprise environments. For decision-makers balancing capability with ROI, it offers a clearer path to deployment without sacrificing performance or safety.
Comments are closed, but trackbacks and pingbacks are open.